Wiebe Van Ranst
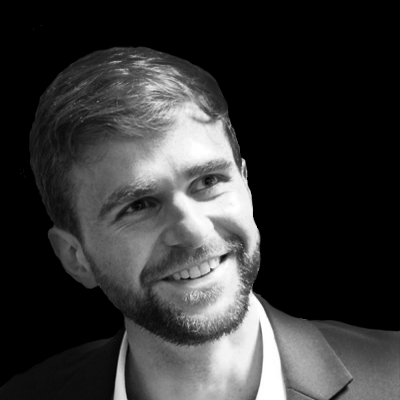
About
Wiebe Van Ranst was born on the 9th of November 1990 in Bornem, Belgium. In 2013 he got his master’s degree in Electronics-ICT. Both Wiebe’s bachelor’s and master’s thesis were about GPU processing which was still a very small field at the time. Starting a PhD was a logical continuation of this. Wiebe started his PhD in 2013, after finishing his master’s thesis, under professor Joost Vennekens. His main interest then mainly focused on artificial intelligence in general and GPU computing. In 2016 Wiebe briefly worked on a start-up company called obtronics, focusing on computer vision, the technology of which was one year later taken over by the company RoboVision. During this experience Wiebe’s interests shifted towards GPU processing for computer vision, and later also computer vision itself. His research was then also supervised by professor Toon Goedemé. Wiebe is currently active as a post-doctoral researcher in the EAVISE research group of KU Leuven. Currently, his research is on applying neural nets on embedded hardware.
Wiebe's talk will be about recent research on real-world adversarial samples.
Talk
Real-world adversarial examples
Level: General
Adversarial attacks on machine learning models have seen increasing interest in the past years. By making only subtle changes to the input of a convolutional neural network, the output of the network can be swayed to output a completely different result. The first attacks did this by changing pixel values of an input image slightly to fool a classifier to output the wrong class. Other approaches have tried to learn "patches" that can be applied to an object to fool detectors and classifiers. Some of these approaches have also shown that these attacks are feasible in the real-world, i.e. by modifying an object and filming it with a video camera. However, all of these approaches target classes that contain almost no intra-class variety (e.g. stop signs). The known structure of the object is then used to generate an adversarial patch on top of it.This talk will be about our work on how to generate adversarial patches to targets with lots of intra-class variety, namely persons. In this work, the goal is to generate a patch that is able successfully hide a person from a person detector. With this goal in mind we explore the possibility of being able to maliciously circumvent surveillance systems.