Miklós Tóth
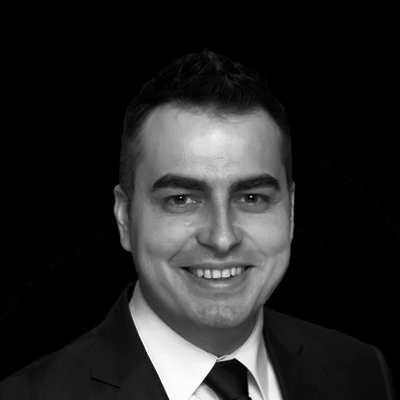
About
Miklos is a senior IT professional with strong business-related skills, he has an entrepreneurial mindset combined with "the Advocate" (INFJ) personality. He started to learn software development when he was 15, therefore he studied Information Technology and Mathematics at University of Szeged. During the 3. semester he founded his first company with other fellow students where they were working during day and night as a garage firm. Later he moved to Germany to work for Audi as a software developer then switched to Volkswagen but remained as an external colleague. Then he spent 1 year in Hungary worked for the German Audi department as an external senior software developer, but he needed new challenges, therefore he accepted a position in Beijing, China at Volkswagen Group China (VGC) as an IT Consultant. Being an expat for 3 years in the capital he managed to create a Software Quality measurement platform for the IT department which controlled the software suppliers of VGC. During this period he started to be interested in data science, machine learning and its business-related opportunities. In 2015 relocated to Hungary and founded his first startup aiwebtesting.com, and started to teach at Audi Academy as an IT Trainer. In the same year he started an MBA degree at CEU (Central European University), he successfully graduated in 2017. He works as a Data Science Trainer, he delivers Big Data and Machine Learning courses at multinational companies and soon he will launch his ML-focused podcast.
Workshop
Small data, great insights: ML/DL tricks with restricted data
Level: General
With all the talk about big data, in practical use cases we very often find ourselves in settings, where specific data is very limited, so we have to resort to special techniques to be able to train reasonably performing models. In this workshop - building on their hands on experience as well as theoretical reflections accumulated during teaching and mentoring - Miklós and Levente demonstrate a wide variety of techniques combatting "small data", and also along the way try to draw some conclusions about the generalization abilities of (deep) machine learning models, as well as demonstrate with hands on exercises the application of these methods. Who is this for: data scientists with practical experience, who would like to broaden the palette of their tools and gain some theoretical insights as well. Prerequisites: Good command of Python and its modeling ecosystem, some experience and knowledge in machine learning and deep learning systems.