Levente Szabados
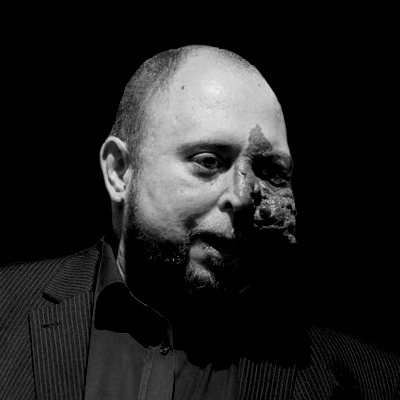
About
Levente is a long time enthusiast of anything cognitive. Originally a programmer, but finished his masters as a Buddhist Theologian, with cognitive science specialization. He started to do AI related work in the early 2000s in knowledge representation, then switched over to become the lead of research for a quantitative finance research group. He later founded an award winning startup (Best Chatbot / AI solution Central European Startup Award, 2016) where he acted as CTO and lead researcher in NLP.
Currently he is teaching at the Frankfurt School of Finance and Management (Deep Learning, NLP and Frontiers of AI classes at the Masters of Data Science, as well as certificate courses), and works as a freelance consultant for a broad range of machine learning projects from pharma to manufacturing.
He is enthusiastic about DL, especially time series and NLP topics, and shares this also in meetups and a podcast.
Workshop
Small data, great insights: ML/DL tricks with restricted data
Level: General
With all the talk about big data, in practical use cases we very often find ourselves in settings, where specific data is very limited, so we have to resort to special techniques to be able to train reasonably performing models. In this workshop - building on their hands on experience as well as theoretical reflections accumulated during teaching and mentoring - Miklós and Levente demonstrate a wide variety of techniques combatting "small data", and also along the way try to draw some conclusions about the generalization abilities of (deep) machine learning models, as well as demonstrate with hands on exercises the application of these methods. Who is this for: data scientists with practical experience, who would like to broaden the palette of their tools and gain some theoretical insights as well. Prerequisites: Good command of Python and its modeling ecosystem, some experience and knowledge in machine learning and deep learning systems.