Jigyasa Grover
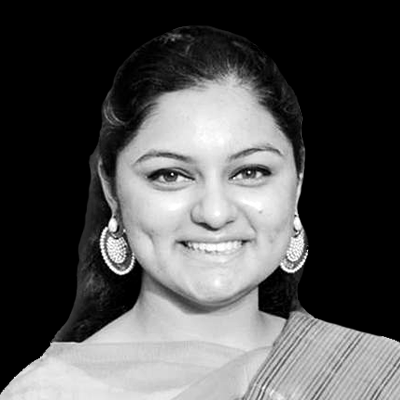
About
Red Hat Women in Open Source Academic Award Winner 2017, Google Summer of Code alumna and currently a Machine Learning Engineer at Twitter Inc., Jigyasa Grover is an ardent open source enthusiast along-with being a budding researcher having worked at San Diego Supercomputer Center, National Research Council of Canada and Institute of Research \u0026 Development France. She has also briefly worked on anomaly detection framework in the ads system at Facebook. A feminist by heart, she was involved as Director of Women Who Code and Lead of Google Women Techmakers for a handful of years to help bridge gender gap in technology. She has delivered keynotes and talks at Open Source 101, Red Hat Summit, University of California San Diego Guest Lecture Series, FOSSASIA OpenTech Summit Singapore, Society of Women Engineers Conference, WWC Regional Leadership Summit India, Grace Hopper Celebration of Women in Computing India, IEEE Women in Engineering International Leadership Summit \u0026 Women Techmakers Meet to name a few. In her quest to build a powerful bunch of girls and boys alike believing in “we rise by lifting others” she mentors aspiring developers in the global programs like Google Summer of Code, Google Code- In, ‘Learn IT, Girl !’
Talk
Fueling Machine Learning with Feature Engineering
Level: General
In the contemporary world of learning algorithms - “data is the new oil”. Data demands efficient refinement to expose valuable information. To lay a strong foundation for the state-of-the-art machine learning algorithms to work their magic, the crude oil-like data needs to be infused with domain knowledge and extracted into “features”. This talk aims to introduce the audience to the subject of Feature Engineering, and talk about the power of the most creative aspect of data science which often does not get its due limelight.It will also walk the audience through the process of feature engineering as done in formal settings with a simple hands-on Pythonic example on publicly available data, along with putting forward some popular techniques like hashing, encoding, and embedding, which assists in pulling the most out of the data after giving it a proper structure for predictive modeling. Terms pertaining to the realm of feature engineering like relevance, selection, combination, and explosion will also be discussed. The goal is to institute the importance of data, especially in its worthy format, and the spell it casts on fabricating smart learning algorithms.