Camille Eddy
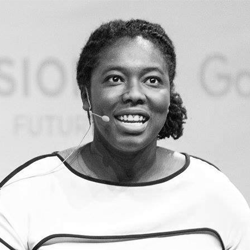
About
Camille Eddy began working on advanced robotics during an internship at HP Inc’s headquarters in Palo Alto, California as a Machine Learning Intern. She continued to intern at X, formerly Google X, in their robotics initiative and also began working with NVIDIA on their autonomous car project. She is currently studying for a Bachelor's degree in Mechanical Engineering. Camille speaks nationally on inclusion in the tech community and regularly writes on the topic. She also enjoys volunteering for STEM advocacy organizations for young girls and students.
It is essential to consider how our products are designed. We will dive deep into several use cases of how the tech industry uses data sets and how often the same data set may be across the tech industry causing bias issues to propagate. Another important aspect of the design is our bias blindspots as human beings and the concept of levers or tools to unveil this bias and create meaningful suggestions on how to seek out clarity and inclusion. Enter explainable AI or fairness AI tools that produce suggestions for analyzing projects. The audience members who will benefit the most from this talk are those with some familiarity with AI tools and general knowledge of common data sets in use today.
Key takeaways:
- How AI has been used in personal data
- How data sets for popular applications have been created
- How often data sets are used across disciplines
- Todays out of the box applications by Google and Microsoft
- Authors and grassroots organizations
Talk
Recognizing cultural bias in AI
Level: General
In a world that is being increasingly run on artificial intelligence do we understand how algorithms make their decisions? Where humans have trouble making the right choices for culture including race, language, accessibility and equality, the algorithms we use should also face the same questions. The purpose of this talk is to walk through everyday examples of services we all use and how they have adapted machine learning to become more inclusive. We will explore what we can do to create culturally sensitive computer intelligence and why that is important for the future of AI.
It is essential to consider how our products are designed. We will dive deep into several use cases of how the tech industry uses data sets and how often the same data set may be across the tech industry causing bias issues to propagate. Another important aspect of the design is our bias blindspots as human beings and the concept of levers or tools to unveil this bias and create meaningful suggestions on how to seek out clarity and inclusion. Enter explainable AI or fairness AI tools that produce suggestions for analyzing projects. The audience members who will benefit the most from this talk are those with some familiarity with AI tools and general knowledge of common data sets in use today.
Key takeaways:
- How AI has been used in personal data
- How data sets for popular applications have been created
- How often data sets are used across disciplines
- Todays out of the box applications by Google and Microsoft
- Authors and grassroots organizations